Nvidia, a GPU producer, has introduced Magic3D, a generative AI that may produce 3D fashions from a textual content immediate.
Generative AI for 3D modeling aids within the conceptualization of elements with complicated, and natural shapes. Whereas, 3D printing, is a perfect expertise for bringing these shapes to life as a result of it’s able to producing complicated buildings whereas additionally being cost-effective.
The outcomes from the annual 3D Printing Business Government Survey, present that the automated era of 3D fashions utilizing AI is a sizzling subject.
Magic3D creates a 3D mesh mannequin with coloured texture inside 40 minutes. This comes proper after the corporate entered a immediate like “A blue poison-dart frog sitting on a water lily.” The outcome obtained, with enhancements, could be utilized in CGI artwork scenes or video video games. Nvidia outlines Magic3D in its tutorial paper as a response to DreamFusion, a text-to-3D mannequin launched by Google researchers in September 2022. In different information, Physna Inc. created a generative AI prototype for 3D fashions and scenes in two weeks utilizing 8,000 fashions.
The researchers within the paper defined how this expertise will enable anybody to create 3D fashions with out the necessity for particular coaching. “As soon as refined, the ensuing expertise may velocity up online game (and VR) improvement and maybe ultimately discover functions in particular results for movie and TV. We hope with Magic3D, we are able to democratize 3D synthesis and open up everybody’s creativity in 3D content material creation.”
Nvidia is well-placed to progress AI. The corporate’s GPUs can create lifelike graphics utilizing shaders, which instruct every pixel in a picture on the right way to show in a particular gentle. The shader is calculated for every pixel, a repetitive calculation throughout quite a few pixels. Nvidia GPUs can shortly render pictures as a result of their design for conducting a number of easy calculations, like shading pixels, without delay, not like Intel microprocessors or general-purpose CPUs. Nvidia sees AI functions as a essential development driver, Bloomberg has attributed a $4.6 billion enhance within the wealth of Nvidia founder Jensen Huang to the recognition of ChatGPT – an AI chatbot.
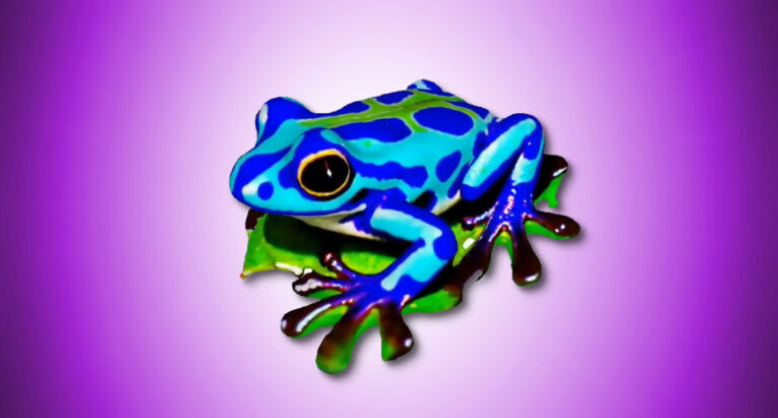
What duties can Magic3D carry out?
Magic3D employs a two-stage methodology that takes a tough mannequin created in low decision and optimizes it to the next decision which is analogous to DreamFusion utilizing a text-to-image mannequin to supply a 2D picture that’s then optimized into volumetric NeRF (Neural radiance subject) information. Primarily based on the authors of the paper, the ensuing Magic3D approach can produce 3D objects twice as quick as DreamFusion.
Magic3D also can conduct prompt-based 3D mesh enhancing. Offered a base immediate and a low-resolution 3D mannequin, the textual content could be modified to vary the ensuing mannequin. Moreover, the authors of Magic3D illustrated preserving the identical topic throughout a number of generations (an idea generally known as coherence) and implementing the model of a 2D picture (equivalent to a cubist portray) to a 3D mannequin.
Generative AI and 3D printing: a future with enormous potential
Paul Powers, Founder, and CEO of Physna Inc. shared his ideas on creating equitable 3D Generative AI. The CEO says that generative AI conquered 2022 and consequently the agency determined to take a dive into combining 3D printing and generative AI. Though Physna is a 3D search and evaluation firm targeted on engineering and design functions in AR/VR and manufacturing, it constructed a really fundamental generative AI prototype for 3D fashions and scenes in 2 weeks utilizing solely 8,000 fashions with simply 3 engineers.
Powers additional defined the explanation behind this experiment. He claims that generative AI has taken many industries by storm however is missing behind in 3D printing. The principle causes behind this delay are difficult 3D fashions and a scarcity of labeled 3D information. 3D fashions have conventionally been tough to create, are available in a wide range of incompatible codecs, and have obtained scant consideration compared to 2D mannequin evaluation (textual content, pictures, video, and so forth.). Few enterprises are fitted to focus on 3D as a result of it has traditionally been a tough subject to beat on the analytical stage.
Moreover, Google’s DreamFusion group summarized the second subject of their article final yr. There may be much less 3D information as in comparison with 2D information. Google’s DreamFusion group utilized NeRFs in the identical manner that Nvidia’s Magic3D group did (Neural Radiance Fields). They’re additionally empty “shells” within the sense that they lack geometry and inner elements, says Paul. This implies customers not solely possess much less data on the item at hand, nevertheless it’s additionally tough to make assumptions concerning the expertise. Whereas coaching on NeRFs could also be extra useful than coaching on 2D fashions, as Google’s DreamFusion group identified, “NeRFs isn’t an excellent substitute for true, labeled 3D fashions.” Which means, within the absence of an answer, generative AI is not going to carry out almost as properly in 3D because it does in different areas within the close to future. The corporate additional carried out some experiments to examine the compatibility of generative AI with 3D printing.
How does GPU computing enhance 3D printing?
GPU computing entails the usage of a GPU (graphics processing unit) as a co-processor to expedite CPUs for technological and scientific computing. By offloading among the time-consuming and compute-intensive code, the GPU hastens CPU-based functions. The rest of the applying continues to run on the CPU. From the person perspective, the applying runs faster because it makes use of the GPU’s parallel processing energy to boost efficiency. The sort of computing is named “hybrid” or “heterogeneous” computing. A CPU usually has 4 to eight CPU cores, whereas a GPU usually has a whole bunch of smaller cores. The GPU features its excessive computing efficiency from its large parallel construction.
Software builders can reap the benefits of the parallel GPU structure’s efficiency by using NVIDIA’s “CUDA” parallel programming mannequin. The NVIDIA CUDA parallel-programming mannequin is supported by all NVIDIA GPUs, together with GeForce, Quadro, and Tesla. Beforehand, Nvidia launched a manner of changing 2D pictures into 3D fashions. The framework demonstrates how it’s doable to deduce form, texture, and lightweight from a single picture, in a way just like how the bare eye works. NVIDIA PR specialist Lauren Finkle wrote on the corporate weblog, “Shut your left eye as you have a look at this display. Now shut your proper eye and open your left, you’ll discover that your visual view shifts relying on which eye you’re utilizing. That’s as a result of whereas we see in two dimensions, the photographs captured by your retinas are mixed to supply depth and produce a way of three-dimensionality.”
The NVIDIA rendering framework, generally known as a differentiable interpolation-based renderer, or DIB-R, has the potential to help and expedite totally different areas of 3D design and robotics, rendering 3D fashions in seconds. In accordance with Finkle, the 3D world we exist in is definitely considered by way of a 2D lens, which is named stereoscopic imaginative and prescient. Depth is created within the mind by merging pictures seen by way of every eye, giving the impression of a three-dimensional picture. DIB-R, which works on an identical precept, can predict the form, colour, texture, and lighting of a picture by remodeling enter from a 2D picture right into a map. This map is then utilized to create a polygon sphere, leading to a 3D mannequin that represents the element within the authentic 2D picture.
Elsewhere, Daghan Cam, beforehand a instructing fellow on the College Faculty London’s Bartlett Faculty of Structure, created a flawless 3D printed structure utilizing GPU computing. Cam used his experience with the CUDA parallel programming mannequin and NVIDIA GPUs to show his robotic fabrication system to make the most of algorithms to complete his abstractly designed buildings earlier than 3D printing a 3D printed prototype. Cam turned to Boston Restricted and Materialise to 3D print his modernistic prototype design after ending the 3D mannequin with a Quadro K6000 graphics card and Tesla K40 GPU accelerator. Materialise’s high-resolution Mammoth stereolithography printer, able to producing large-scale and sophisticated prints in a single piece, was employed to 3D print the prototype. The finished prototype was intricate, summary, and intensely pleasing to the attention, and gave the impression to be completely fitted to show on the MOMA or the Louvre.
Characteristic picture exhibits a poison dart frog rendered as a 3D mannequin by Magic3D. Picture through Nvidia.
What does the way forward for 3D printing maintain?
Did the consultants get the tendencies in 3D printing for final yr proper?
To remain updated with the most recent 3D printing information, don’t neglect to subscribe to the 3D Printing Business publication or observe us on Twitter, or like our web page on Fb.
Whilst you’re right here, why not subscribe to our Youtube channel? That includes dialogue, debriefs, video shorts, and webinar replays.
Are you in search of a job within the additive manufacturing trade? Go to 3D Printing Jobs for a collection of roles within the trade.